Beyond cat food and guitar picks: how AI could make marketing more human
The first thing most people do when they sit down with a Large Language Model (LLM), like ChatGPT is to ask it to write them something – maybe it’s a poem in French or some gangster rap as if written by Shakespeare. Cute. Very quickly, they move to asking it to tackle a work-related task: write me a promotional email, or some ad copy. And the emotion shifts from cute to OMG. But as impressive as that is, I think many of today’s AI use cases are missing a bigger customer opportunity – one that will build brand loyalty through a new kind of customer experience.
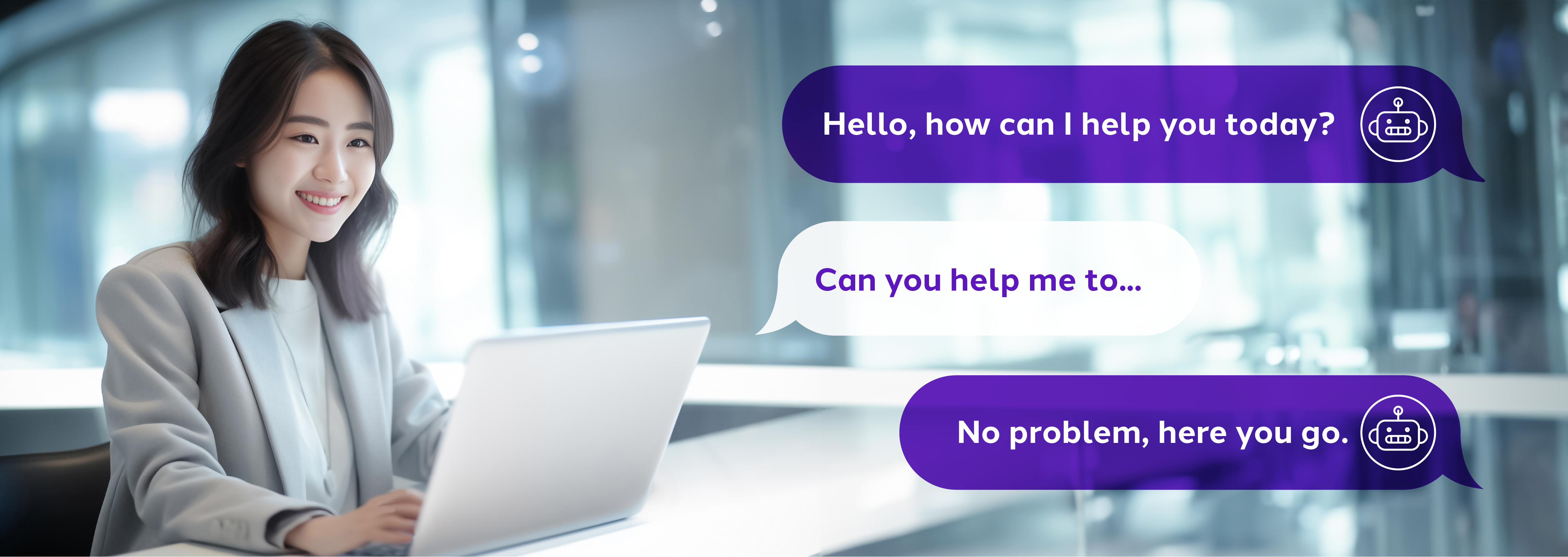
The first round of marketing use-cases for generative AI are ultimately about cost savings. An automated chatbot might save the need for humans at the other end of the line. Maybe we need fewer copywriters in the future, or maybe we can accelerate the creative process. Maybe that will lead to better creative outcomes, but ultimately it is more likely to save cost, than to drive growth.
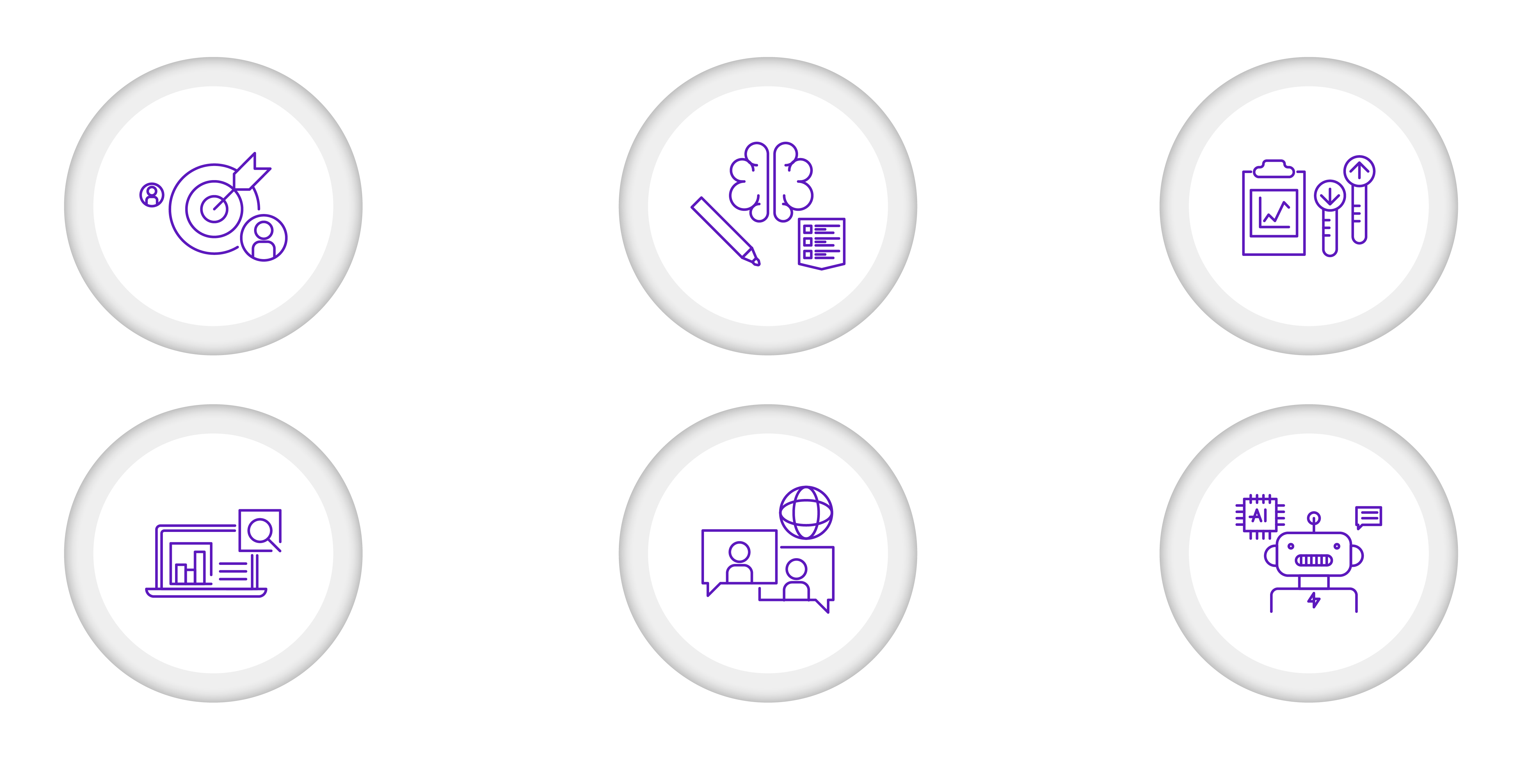
The second round of marketing use-cases talk about personalisation at scale. Now we start to get into more of an opportunity to drive engagement and revenue, but arguably it’s still a cost play. It’s an important cost play, because the road to segment-of-one personalisation is a very expensive and automation is the only feasible way to achieve it. But on the revenue side, I believe there is currently only an incremental revenue opportunity from personalisation. Why? Because for all the squillions of dollars invested in Customer Data Platforms (CDPs) and data scientists to mine those platforms, we still know very little about our customers. The problem stems from our attitude towards data collection.
CDPs contain mostly transactional or technical engagement data: what you bought, what you put in your cart, what you hovered your mouse over. This is all useful data, but at the end of the day, most of the “insights” that come out of them are “if…then” insights, such as “if a person has a cat, they are likely to buy cat food” or “if someone has recently bought a guitar, they may be interested in guitar picks”. Maybe they contain some basic demographic information, or even your birthday (so when your special day rolls around, they can offer you 40% off cat-food and guitar picks) but they know nothing about why you buy what you buy or how you use it.
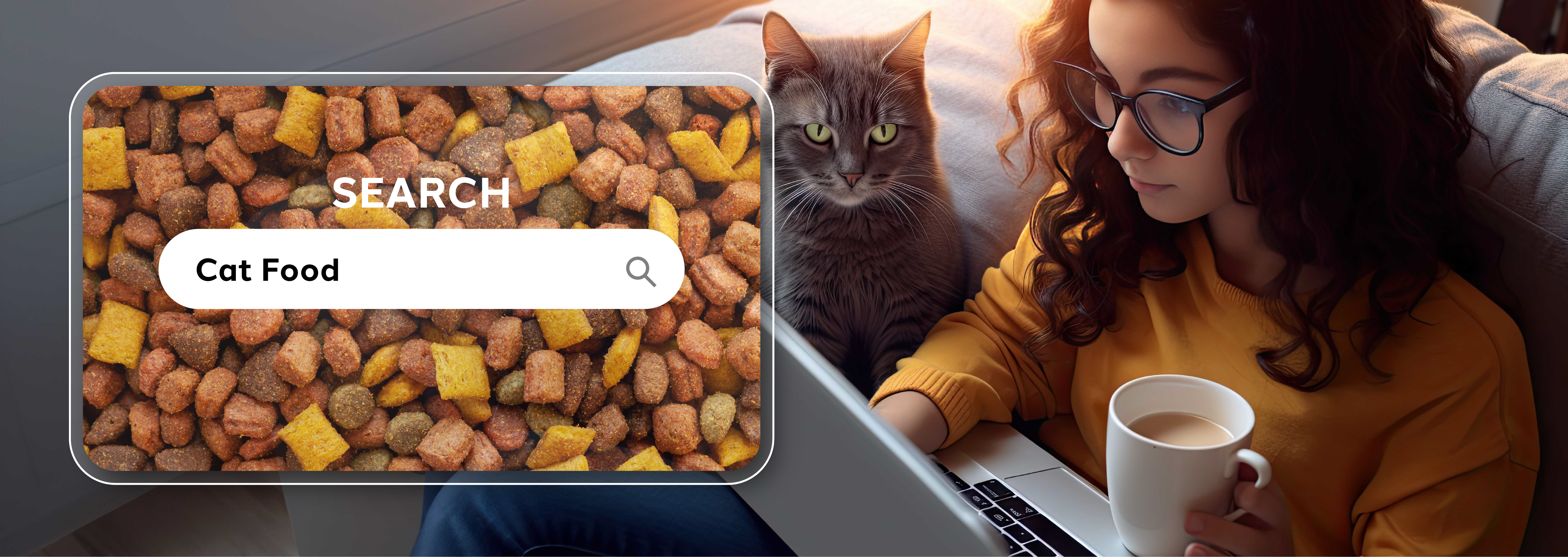
Leading companies have built massive predictive models that can detect patterns and infer intent, but they are still guessing and when they get the result wrong (and often even when they get it right) it can be unsettling to customers. So, in the short term, generative AI is of limited (i.e. positive, but incremental) use for personalisation.
But the good news is, there is an immediate opportunity to use the same technologies that sit behind ChatGPT to better understand our customers and, ultimately, to build deeper, personal, and trusted relationships with them. LLMs offer an unprecedented opportunity to ingest customer input and conversations at scale and convert them into vastly more nuanced and actionable structured data-sets.
Imagine you’re an e-commerce retailer with a popular, but expensive human-based chat option. Option 1 is to replace them with a GPT-powered model. Perhaps the responses get a little more generic, or even a little better, but ultimately the impact is the cost savings. Option 2 is to feed your years of conversation logs and develop a systematic and actionable view of what your customers care about or are concerned and confused by. Ultimately you might still replace the humans, but in the immediate term, building that understanding into a rich and actionable database is vastly more valuable.
In the example(s) above, customers were potentially told their conversation would be recorded for training purposes (does that extend model training?) but it would likely be unethical to use the learnings from it to market directly to individuals. Still, it would be incredibly useful for identifying broader trends in the WHY and HOW people think about and interact with the brand.
From there, the opportunity is to invent new channels to conduct customer conversations and design a data-value exchange where customers are encouraged to share not only WHAT the like, but WHY they like it and HOW they shop for it and use it.
"Aside: For an excellent primer on the data-value-exchange and trust, read this ever-relevant article from dentsu's 2022 Year in Review, or fully arm yourself by delving in our APAC data consciousness project".
With this, brands can deliver vastly more relevant recommendations, which when delivered transparently have real value to customers. While these types of recommendations are based on personal information, it’s personal information that customers readily share because they know and understand the value they will get in return.
Generative AI has the potential to disrupt so many things. Deep fakes are already disrupting the trust we have in what we see, hear, and read. Bots in the retail industry, by crawling sites and providing product and price recommendations outside of retailer ecosystems, are disrupting the traditional curation value proposition. And yes, the way we do our jobs (and many jobs themselves) will be disrupted as processes get automated. But ironically, this technology also offers an opportunity for redemption: by understand sentiment and having personalised real conversations at scale, we can generate new value for customers, building trust, creating unmatchable customer experiences, and ultimately making our interactions feel more serendipitous, more intimate, more human.
Contact us
Leave us your details and a member of our team will get back to you as soon as possible.
Thank you!
Your details were submitted successfully.
There was a problem!
It seems there was an error submitting your details. Please try again later.